Unified intelligence and data engineering
Gaining the competitive edge using unified intelligence and data engineering
In today's rapidly evolving consumer business landscape, the ability to harness and leverage data effectively has become a crucial determinant of success. There is intense competition for retailers and consumer packaged goods (CPG) companies to get closer to their end-consumers. As organizations strive to meet growing customer expectations and stay competitive, advanced technologies such as generative AI (genAI) are emerging as key enablers. However, to truly unlock the potential of these technologies, organizations must go beyond traditional data silos to embrace a more integrated approach to data management and analysis. This is where unified intelligence and data engineering come into play. In the absence of unified intelligence, the system cannot provide a holistic view of what is happening across the business. This lack of integration can delay decisions related to emerging challenges and opportunities.
Breaking down silos
Traditionally, consumer businesses have operated with data scattered across various departments and systems. Sales, marketing, supply chain, and customer service often maintain their own datasets, leading to fragmented and inconsistent information. These functions usually have point solutions that can answer a few questions and address a few problems but cannot adequately address the complexities that large retailers and CPG organizations face. This siloed approach hinders the ability to gain comprehensive insights and make informed decisions.
Unified intelligence addresses this challenge by integrating and harmonizing data from diverse and disparate sources, creating a single, cohesive view of the business. This integration enables organizations to see the bigger picture, understand the interconnectedness of their operations, and make strategic decisions based on holistic insights.
Breaking down data silos is no longer just a technical challenge—it is a strategic imperative. In today's data-driven world, seamless data integration across all departments is essential for unlocking the full potential of advanced analytics and delivering a cohesive customer experience. Retailers and CPG firms must prioritize unifying their data assets to stay competitive and truly understand their customers.
– Amita Mirajkar, Head of Data Management for CPGR Industry, EXL
Challenges to unified intelligence
As organizations undertake consumer-centric initiatives using data, navigating the complexities of managing data at scale emerges as a formidable obstacle for AI and advanced analytics. The proliferation of data sources exacerbates this challenge, drawing from an array of sources such as point of sale (POS) systems, customer databases, online platforms, third-party providers, customer interactions, social media, supply chain logistics, and Internet of Things (IoT) sensors within stores. Integrating these diverse data sources is often challenging. However, if done successfully, it can enable data-driven decisions across the enterprise.
Retail and CPG companies are tackling this challenge by using data lakes to store structured and unstructured data at scale. Leveraging and integrating external market and consumer data can fill in gaps and provide a complete view. For instance, an electronics retailer can combine tech trends with sales data to understand how a new smartphone launch will affect accessory sales. This connected data reveals the accessories to prioritize, the optimal promotion type and timing, as well as inventory demands based on consumer reviews. These insights enable data-driven decisions to improve product assortment and marketing, enhancing customer satisfaction and profitability.
Other challenges include:
- Ensuring data quality and establishing a data governance framework: In a 2023 survey involving 334 CDOs and data leaders from various organizations (sponsored by Amazon Web Services and the MIT Chief Data Officer/Information Quality Symposium), 46% identified “data quality” as the primary challenge in harnessing genAI’s potential within their organizations. This underscores the need for significant changes in data strategy and emphasizes the need for rigorous data quality checks to maintain data reliability. Additionally, establishing a robust data governance framework with clear data ownership, access controls, and compliance with regulations, such as GDPR and CCPA, is essential to ensure data security and trustworthiness.
Retail and CPG companies are addressing this challenge by defining clear data ownership roles, implementing advanced access controls, and adopting automated compliance tools to meet GDPR and CCPA requirements. They are also establishing data stewardship programs and integrating AI and machine learning (ML) to enhance data quality and detect anomalies. Cross-functional collaboration is key to aligning data governance policies with business objectives, while transparency initiatives help build consumer trust in data privacy practices. These trends reflect a shift towards a more structured and proactive approach to data governance in the industry.
- Data engineering complexity: Creating a unified view of data through metadata management (MDM) is a complex task that requires consolidating disparate data sources into a single source of truth. MDM is essential for enriching data with details about its origin, structure, and usage, adding another layer of complexity to data engineering. A recent MDM survey from McKinsey involving 80 large global organizations outlined four top objectives for maturing MDM capabilities: improving customer experience and satisfaction, enhancing revenue growth through better cross- and up-selling opportunities, increasing sales productivity, and streamlining reporting.
The trend is towards implementing customizable MDM solutions that enable businesses to design data models, workflows, and user interfaces tailored to their specific processes and goals. This includes setting data validation rules, stewardship workflows, and quality standards. While generic solutions may offer a quick start, they often fail to meet the complex and unique needs of individual organizations. Customized MDM ensures that data is accurate, consistent, and reliable, ultimately enhancing decision-making and supporting strategic initiatives.
- Leveraging advanced analytics: Advanced analytics can help retailers and brands derive valuable insights from historical data. A 2023 Salesforce survey involving 14,300 consumers and business buyers highlights that 76% of customers expect companies to understand their needs and expectations. Consumer businesses that analyze past trends, identify current trends, and accurately predict future outcomes prevail. Retail and CPG companies are increasingly their use of machine learning to drive customer segmentation and achieve precise targeting and hyper-personalized marketing strategies, which are essential for enhancing customer engagement and satisfaction. Advanced analytics is also being employed to optimize inventory through predictive management and implement dynamic pricing strategies. Additionally, these companies are integrating connected commerce experiences and using analytics for churn prediction and operational efficiency. Sentiment analysis and social listening/meaning further inform product development and brand strategy, fostering data-driven decision-making across the sector. These advancements collectively drive a more responsive and efficient Retail CPG landscape.
- Developing scalable AI and machine learning infrastructure: Obsolete or inadequate technology can hinder the smooth integration and scalability of AI systems, preventing organizations from fully capitalizing on AI's potential benefits. For consumer businesses to succeed, it is essential to build scalable AI models that manage vast amounts of operational data. What is more, continuous training and validation of these models with fresh data will help maintain their accuracy and relevance. Companies that employ scalable AI models are more readily able to adapt to changing market conditions, talent gaps, and consumer behaviors and seize a competitive advantage.
Retail and CPG companies are increasingly investing in scalable AI and ML infrastructures to enhance personalization, optimize supply chains, and automate processes across the value chain. Key trends include real-time data processing, advanced customer insights, and cloud-based solutions for flexibility and cost-efficiency.
Make the transition easier
As disjointed systems become relics of the past, unified intelligent systems are becoming more prevalent to support day-to-day operations. The time required for a full transition depends on the scale and complexity of the operations. Typical stages of maturity for Retail and CPG companies are as follows:
- Early stage: At this stage, companies are just beginning and often struggle with siloed data and functions. The focus here is on establishing the data strategy with shift-left approach for data quality and governance, ensuring the secure handling of personal and transaction data in compliance with regulations. Building a solid foundation for data management here is crucial.
- Intermediate stage: Companies in this stage start integrating a few functions, particularly focusing on monetizing first-party customer data and creating a unified customer view. They shift from traditional campaigns to rapid experimentation and agile execution, aiming to enhance their data-driven marketing strategies.
- Advanced stage: At this level, companies address integration challenges with logistics partners and third-party data providers. They emphasize creating cohesive and connected experiences by leveraging AI, real-time data analytics, and adhering to industry data governance standards, thus enhancing operational efficiency and customer satisfaction.
- Progressive strategy: Organizations at this stage implement cutting-edge technologies to craft personalized, efficient, and superior connected experiences. They focus on enhancing insights from the consumer journey, using advanced analytics to drive strategic decisions and deliver exceptional customer engagement.
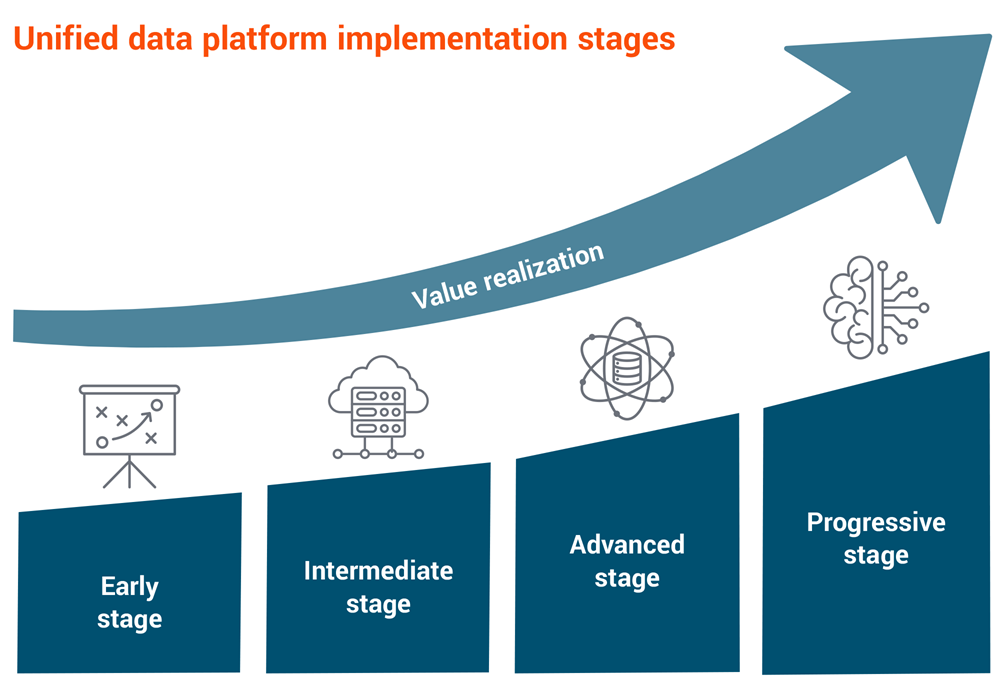
Streamlining data management and AI deployment with EXL’s unified intelligence solutions
EXL offers end-to-end solutions for managing data at scale, essential for effective AI and advanced analytics. Our unified intelligence approach, which spans all key technology stacks for data management and cloud enablement, accelerates the development of a customized MDM system.
We ensure the seamless integration of diverse data sources, enabling data-driven decisions across the business. Our services include robust data quality and governance frameworks that ensure compliance with regulations like GDPR. We also simplify data engineering through customizable MDM solutions, enriching data and streamlining operations. Additionally, we help develop scalable AI models that adapt to evolving market conditions, giving companies a competitive edge while building on the maturing of their unified intelligence systems.
Learn more now!
To learn more about unified intelligence systems in retail, or to speak to one of our experts on the topic, please visit EXLservice.com.